Disparate data integration case for connected factories using timestamps
Abstract
Manufacturing data integration of machine, process, and sensor data from the shop floor remains an important issue to achieve the anticipated business value of fully connected factories. Integrated manufacturing data has been a hallmark of Industry 4.0 initiatives, because integrated data precipitates better decision-making for cost, schedule, and system optimizations. In this paper, we extend work on optimizing manufacturing costs, describing an algorithm using timestamps to integrate previously unassociated quality and test information, enabling us to better identify and eliminate redundant tests. Results are provided and discussed, and we suggest the approach described may be valuable for some types of heterogeneous manufacturing data integration where timestamps and event chronologies are available.
Keywords
Full Text:
PDFReferences
References
Berryman S, Brio A, Burkhardt A, Gharbiah H, Hubner G, Woudenberg M, et al. Concept of Operations for Test Cost Analytics Teams in Complex Manufacturing Environments. In IEEE AutoTestCon Conference; 2017; Schaumburg, IL.
Burkhardt A, Berryman S, Brio A, Ferkau S, Hubner G, Lynch K, et al. Measuring Manufacturing Test Data Analysis Quality Costs. In IEEE AutoTestCon Conference; 2018; National Harbor.
Maksi L, Berryman S, Brio A, Burkhardt A, Elder S, Ferkau S, et al. Study on Deriving Common Factory Test Platform Requirements Using Historical Test Data. Advanced Aspects of Engineering Research. 2021; 6: 38-49.
Weigel AL. Spacecraft system-level integration and test discrepancies: characterizing distributions and costs. 2000 June.
Jiao J, Tseng MM, Ma Q, Zou Y. Generic bill-of-materials-and-operations for high-variety production management. Concurrent Engineering. 2000; 8(4): 297-321.
Bhat P, Berryman S, Burkhardt A, Cho M, Ferkau S, Gharbiah H, et al. Manufacturing Bill-of-Materials Plus Operations Visualization Using D3. Journal of Information Technology and Software Engineering. 2020 December; 11(1).
Ferkau S, Risch Q, Bhat P, Lynch K. Optimizing Test and Inspection Operations in Complex Engineering Products. Journal of Operations Management and Information Systems. 2021 March; 6(1).
Deming WE. Out Of The Crisis Cambridge: The MIT Press; 1982.
Ohno T. Toyota production system: beyond large-scale production: CRC Press; 1988.
Chahal V, Narwal M. Impact of lean strategies on different industrial lean wastes. International Journal of Theoretical and Applied Mechanics. 2017; 12(2): 275-286.
Lu Y. Industry 4.0: A survey on technologies, applications and open research issues. Journal of industrial information integration. 2017; 6: 1-10.
Colombo AW, Karnouskos S, Kaynak O, Shi Y, Yin S. Industrial cyberphysical systems: A backbone of the fourth industrial revolution. IEEE Industrial Electronics Magazine. 2017; 11(1): 6-16.
Monostori L, Kádár B, Bauernhansl T, Kondoh S, Kumara S, Reinhart G, et al. Cyber-physical systems in manufacturing. CIRP Annals. 2016; 65(2): 621-641.
Lee J, Bagheri B, Kao HA. A cyber-physical systems architecture for industry 4.0-based manufacturing systems. Manufacturing letters. 2015 January; 3: 18-23.
Coronado PDU, Lynn R, Louhichi W, Parto M, Wescoat E, Kurfess T. Part data integration in the Shop Floor Digital Twin: Mobile and cloud technologies to enable a manufacturing execution system. Journal of manufacturing systems. 2018; 48: 25-33.
Tao F, Qi Q, Wang L, Nee AYC. Digital twins and cyber–physical systems toward smart manufacturing and industry 4.0: Correlation and comparison. Engineering. 2019; 5(4): 653-661.
Liu M, Fang S, Dong H, Xu C. Review of digital twin about concepts, technologies, and industrial applications. Journal of Manufacturing Systems. 2021;(58): 346-361.
Hirvonen MS. Streamlining manufacturing data integration. Master's Thesis. Tampere University of Technology; 2017.
Kusiak A. Smart manufacturing must embrace big data. Nature. 2017; 544(7648): 23-25.
Csalódi R, Süle Z, Jaskó S, Holczinger T, Abonyi J. Industry 4.0-driven development of optimization algorithms: A systematic overview. Complexity. 2021: 1-22.
Lim KYH, Zheng P, Chen CH, Huang L. A digital twin-enhanced system for engineering product family design and optimization. Journal of Manufacturing Systems. 2020;(57): 82-93.
Fernandez-Viagas V, Framinan JM. Exploring the benefits of scheduling with advanced and real-time information integration in Industry 4.0: A computational study. Journal of Industrial Information Integration. 2022; 27: 100281.
Jiang Y, Yin S, Li K, Luo H, Kaynak O. Industrial applications of digital twins. Philosophical Transactions of the Royal Society A. 2021; 379(2207): 20200360.
Qi Q, Tao F. Digital Twin and Big Data Towards Smart Manufacturing and Industry 4.0: 360 Degree Comparison. IEEE Access. 2018; 6: 3585-3593.
Zhuang C, Gong J, Liu J. Digital twin-based assembly data management and process traceability for complex products. Journal of manufacturing systems. 2021;(58): 118-131.
Motro A, Anokhin P. Fusionplex: resolution of data inconsistencies in the integration of heterogeneous information sources. Information fusion. 2006; 7(2): 176-196.
Kumbhar M, Ng AH, Bandaru S. A digital twin based framework for detection, diagnosis, and improvement of throughput bottlenecks. Journal of manufacturing systems. 2023;(66): 92-106.
Mayr M, Luftensteiner S, Chasparis GC. Abstracting process mining event logs from process-state data to monitor control-flow of industrial manufacturing processes. 2022;(200): 1442-1450.
Zhu H, Madnick SE, Siegel MD. Effective data integration in the presence of temporal semantic conflicts. 11th International Symposium on Temporal Representation and Reasoning. 2004;: 109-114.
Kritzinger W, Karner M, Traar G, Henjes J, Sihn W. Digital Twin in manufacturing: A categorical literature review and classification. Ifac-PapersOnline. 2018; 51(11): 1016-1022.
Strong DM, Volkoff O. Data Quality Issues in Integrated Enterprise Systems. In MIT International Conference on Information Quality; 2005.
Jones-Farmer LA, Ezell JD, Hazen BT. Applying control chart methods to enhance data quality. Technometrics. 2014 May; 56(1): 29-41.
Degbotse A, Ang AK, Vuong NQ, Tan JS. Predictive analytics in reverse supply chain management commodity life expectancy for quality engineering. In IEEE 19th Electronics Packaging Technology Conference (EPTC); 2017. p. 1-7.
Foley AT. Data quality and reliability analysis of US Marine Corps ground vehicle maintenance records. 2015 June 1.
Mora E, Gaiardelli P, Resta B, Powell D. Exploiting lean benefits through smart manufacturing: A comprehensive perspective. In Lödding H,RR,TK,vCG,KD. IFIP Advances in Information and Communication Technology: Advances in Production Management Systems. The Path to Intelligent, Collaborative and Sustainable Manufacturing. Hamburg: Springer International Publishing; 2017. p. 127-134.
DOI: https://doi.org/10.23954/osj.v8i2.3395
Refbacks
- There are currently no refbacks.

This work is licensed under a Creative Commons Attribution 4.0 International License.
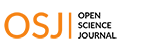
Open Science Journal (OSJ) is multidisciplinary Open Access journal. We accept scientifically rigorous research, regardless of novelty. OSJ broad scope provides a platform to publish original research in all areas of sciences, including interdisciplinary and replication studies as well as negative results.