Winter beans: the use of an unmanned aerial vehicle for monitoring and prediction of crop performance
Abstract
Traditional field-based techniques for phenotyping of crops are based on visual assessment which are subjective and time consuming. A high throughput automated technique using an unmanned aerial vehicle (UAV) with a multispectral image (MSI) camera was used to investigate the correlation between markers of winter bean crop development with eventual crop yield. A simplified approach has been developed using different vegetation indices i.e. NDVI, GNDVI and NDRE, coupled with an iso-cluster classification method to monitor plant characteristics across all growing stages. The UAV-MSI data could then be incorporated into a yield estimator model to estimate the winter bean seed yield. The NDVI approach showed the greatest correlation between the modelled seed yield and the actual seed yield determined on two separate occasions (R2 = 0.84 and R2 = 0.87). In addition, GNDVI and NDRE were a better estimator of seed yield for areas with dense vegetation. These are hence shown to be able to monitor a winter bean harvest in an efficient and timely manner.
Keywords
Full Text:
PDFReferences
LGSeedUK,https://www.arablescotland.org.uk/sites/www.arablescotland.org.uk/files/2020-06/Tundra%20Winter%20Bean%20Technical%20Summary.pdf, (accessed September 2022).
Senova,https://irp.cdnwebsite.com/55da3d94/files/uploaded/Winter%20Bean%20Growers%20Guide%20060422.pdf, (accessed September 2022).
Nickerson, https://www.nickersonseeds.co.uk/products/pulses/tundra/data-sheet/, (accessed September 2022).
Farmers Weekly, Disease alert for winter beans after mild winter - Farmers Weekly (fwi.co.uk) (accessed September 2022).
GrowNotes, https://grdc.com.au/resources-and-publications/grownotes/crop-agronomy/faba-bean-southern-region-grownotes/GrowNote-Faba-South-5-Plant-Growth-Physiology.pdf, (accessed September 2022).
Jimenez-Berni JA, Deery DM, Rozas-Larraondo P, Condon ATG, Rebetzke GJ, James RA, et al. High Throughput Determination of Plant Height, Ground Cover, and Above-Ground Biomass in Wheat with LiDAR. Front Plant Sci. 2018;9:237. doi:10.3389/fpls.2018.00237
Mulla DJ. Twenty five years of remote sensing in precision agriculture: Key advances and remaining knowledge gaps. Biosystems Engineering. 2013;114(4):358-71. doi:10.1016/j.biosystemseng.2012.08.009
Sishodia RP, Ray RL, Singh SK. Applications of Remote Sensing in Precision Agriculture: A Review. Remote Sensing. 2020;12(19). doi:10.3390/rs12193136
Ji Y, Chen Z, Cheng Q, Liu R, Li M, Yan X, et al. Estimation of plant height and yield based on UAV imagery in faba bean (Vicia faba L.). Plant Methods. 2022;18(1):26. doi:10.1186/s13007-022-00861-7
Alabi TR, Abebe AT, Chigeza G, Fowobaje KR. Estimation of soybean grain yield from multispectral high-resolution UAV data with machine learning models in West Africa. Remote Sensing Applications: Society and Environment. 2022;27. doi:10.1016/j.rsase.2022.100782
Maimaitijiang M, Sagan V, Sidike P, Hartling S, Esposito F, Fritschi FB. Soybean yield prediction from UAV using multimodal data fusion and deep learning. Remote Sensing of Environment. 2020;237. doi:10.1016/j.rse.2019.111599
Boiarskii B. Comparison of NDVI and NDRE Indices to Detect Differences in Vegetation and Chlorophyll Content. Journal of Mechanics of Continua and Mathematical Sciences. 2019;spl1(4). doi:10.26782/jmcms.spl.4/2019.11.00003
Shirsath PB, Sehgal VK, Aggarwal PK. Downscaling regional crop yields to local scale using remote sensing. Agriculture. 2020;10(58):2-14. doi:10.3390/agriculture10030058
Xie T, Li J, Yang C, Jiang Z, Chen Y, Guo L, et al. Crop height estimation based on UAV images: Methods, errors, and strategies. Computers and Electronics in Agriculture. 2021;185. doi:10.1016/j.compag.2021.106155
Rouse Jr JW, Haas RH, Schell JA, Deering DW. Monitoring vegetation systems in the Great Plains with ERTS. Third Earth Resources Technology Satellite-1 Symposium: Volume 1; Technical presentations, section B, SC Freden, EP Mercanti, and MA Becker, Eds., NASA Special Publ. InConference Proceedings, Document ID: 19740022592 1974 (pp. 1-9).
Gitelson AA, Kaufman YJ, Merzlyak MN. Use of a green channel in remote sensing of global vegetation from EOS-MODIS. Remote sensing of Environment. 1996 Dec 1;58(3):289-98. https://doi.org/10.1016/S0034-4257(96)00072-7
Sims DA, Gamon JA. Relationships between leaf pigment content and spectral reflectance across a wide range of species, leaf structures and developmental stages. Remote sensing of environment. 2002 Aug 1;81(2-3):337-54. http://dx.doi.org/10.1016/S0034-4257(02)00010-X
DOI: https://doi.org/10.23954/osj.v8i2.3336
Refbacks
- There are currently no refbacks.

This work is licensed under a Creative Commons Attribution 4.0 International License.
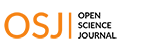
Open Science Journal (OSJ) is multidisciplinary Open Access journal. We accept scientifically rigorous research, regardless of novelty. OSJ broad scope provides a platform to publish original research in all areas of sciences, including interdisciplinary and replication studies as well as negative results.